How reinsurance underwriters should use data, analytics and AI
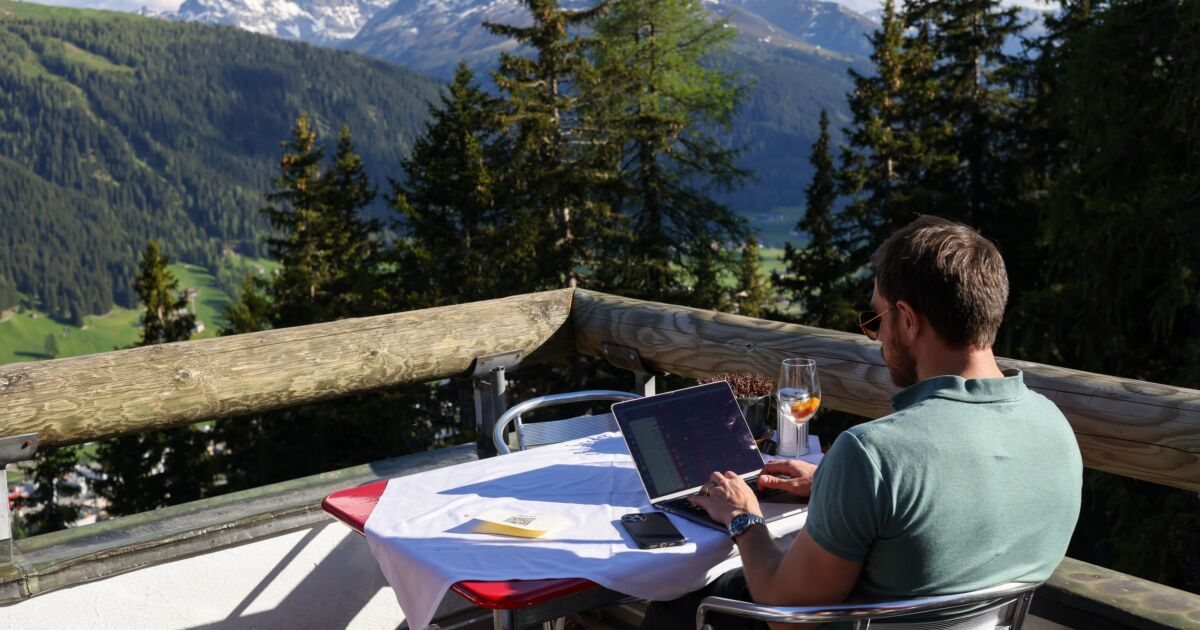
The firming reinsurance market creates a significant opportunity for reinsurers to achieve faster growth.
The reinsurers who can gain better insights from data and translate them into a pricing advantage, powered by automated and streamlined processes enabling faster response to the market will see significant benefits in driving profitable growth. Hence, it is critical for reinsurance carriers to enhance underwriting capabilities by leveraging advantaged technologies with an integrated data strategy, optimized processes and effective utilization of underwriting talent.
The reinsurance industry has made advancements around catastrophe modeling, improving the science around natural hazards, and increasing computational capability, which is helping carriers to develop strong underwriting guidelines and frameworks for underwriters or those authorized to write business. However, still, there is a significant challenge in effectively operationalizing underwriting guidelines in a day-to-day underwriting operation due to data and technology constraints. They have huge amounts of data to assess each risk, but underwriters still struggle to leverage the data effectively.
To arrive at a correct pricing decision, reinsurers need to upgrade their data management and governance processes to ensure data integrity and data quality and develop better methods for integrating data —both internal and external sources — to increase the granularity and speed of the risk assessment, and effective pricing in adherence to the underwriting guidelines.
Once the reinsurers have a solid data foundation, automated workflows can then be added to eliminate manual data consolidation and extraction. Even before reinsurers use the data they have, they have got to input it into their systems — and they mainly rely on outdated methods to do it, manually keying in both structured and unstructured data sets, often several times. Artificial intelligence and machine learning systems are designed exactly for this kind of situation. They can be trained to understand, input, and analyze data, improving both accuracy and speed. This frees highly specialized reinsurance underwriters to focus on more profitable business and building relationships with brokers and customers rather than the back-office operation work.
Many reinsurers still need help with basics such as process design, creating workflows that streamline data and operations, redefining the role of the underwriters, building the right targeting operating models, and putting in the right controls.
Let me share a couple examples that show how integrated data, automation, and AI combine to unleash underwriting expertise.
One multi-line specialty reinsurance carrier we worked with had a fragmented submission management process with multiple systems and data repositories for each line of business. That has resulted in an inefficient underwriting process with highly manual work of accessing multiple sources to gather data, limited validation, poor data quality, etc. The process also lacked transparency of submission in real-time due to system constraints. The key to fixing the carrier’s issues was to streamline the end-to-end process across submissions, risk clearance, risk assessment, quote processing, and policy issuance, and implement a unified workbench for treaty and facultative business across all lines and geographies. A holistic approach to people, process, data, and technology design enabled complex underwriting and ensured UW discipline. Making these changes reduced submission handling time by 70%, decreased underwriting operation costs by 40%, and improved the quote-to-bind ratio resulting in top-line growth and improved broker experience.
We also worked with a reinsurer that was struggling with outdated and inefficient processes that produced inconsistent data. Plus, it needed constant support from the IT department to make changes to existing processes. All these issues created poor customer experiences, impacted productivity, and reduced profitability. Our solution ‘listened’ for the arrival of data files in real-time, extracting meaningful data immediately, accurately, and created new business rules for automated processing. After a short 10-week pilot period, the new system established a better customer and employee experience, improved the accuracy of data conversion, increased translation capabilities by 8x, and reduced the support needed from IT since the business team could make changes five times faster than before.
How should companies proceed?
Reimagine technology-enabled business processes by focusing rigorously on business outcomes and harnessing the data effectively through a purpose-built enterprise analytics insights engine, which then should be scaled and sustained through the right organizational structure.
With the world only becoming more complex, any solution will require an integration of technology and expertise. One smart strategy would be for companies to look for a partner that brings both deep industry knowledge as well as technical chops. A partner can help you navigate a fast-changing business environment while incorporating technological advances.
But the key point is to start as soon as possible. Periods of technology transformation always uplift entire industries — but within those industries, there are always winners and losers. The winners usually start early.