How deep learning could solve business problems
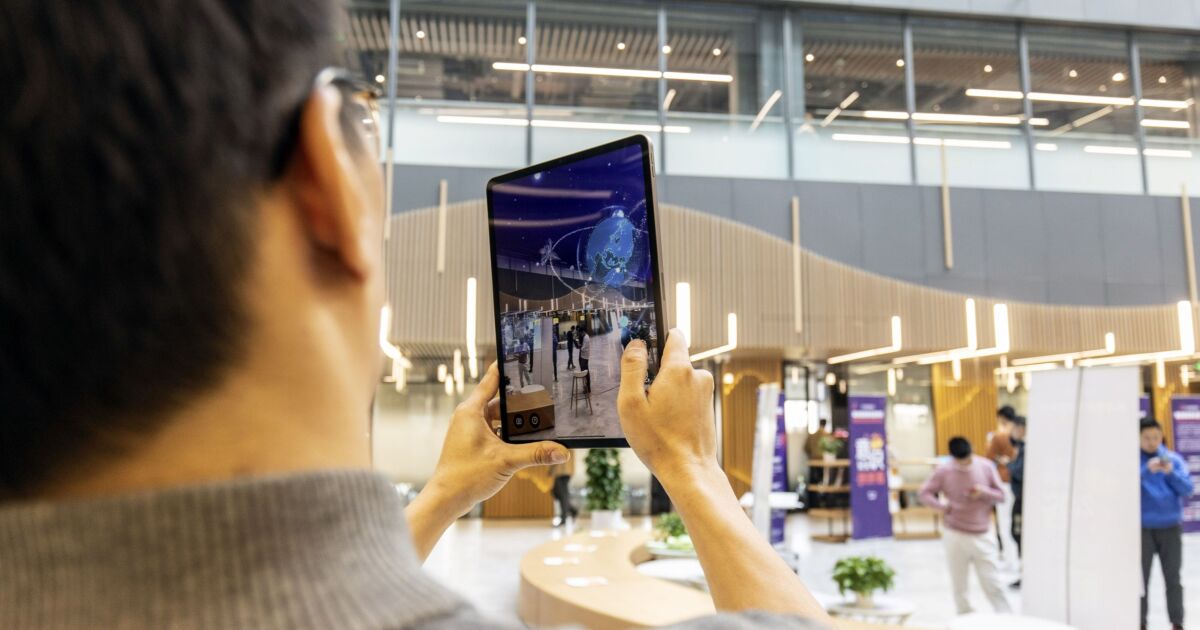
Deep learning signifies the application of very large neural networks to vast amounts of data associated with complex problems. Whereas neural networks have been somewhat constrained in the past by computing power and data availability, advancements in both areas now enable neural networks to be constructed with exponentially more layers and nodes. This enables neural networks to refine their modeling of a problem and create more accurate solutions.
One example of a typical deep learning application is pattern recognition applied to digital images. Simple neural networks might ‘look’ at thousands of images of cats and assess the presence of eyes, ears, whiskers and fur as well as the shapes, sizes and textures of each. The resulting model could become very good at determining whether an image is of a cat or not. A deep neural network would ‘look’ at millions of images and build complex features that describe every small detail of the cat (length of whiskers relative to head size, size and shape of eyes, their location relative to ears and nose, etc.) to form its predictions. Not only could such a model determine cat vs. non-cat much more accurately than the simple model, but it could also potentially predict breed, sub-breed, age, etc. of the cat too.
Any problem where this level of “reasoning” is necessary to make accurate predictions is a good use of deep learning. In more practical terms, think of assessing thousands of complex documents to determine the best course of treatment for a patient. Another example could be the continuous review of financial transactions, news stories, stock prices, and other inputs to identify potentially illegal trading behaviors.
Any business that has massive data sets, large capacity computers, access to highly skilled data scientists, and complex problems to solve can take advantage of deep learning. Healthcare, insurance, banking, investment and e-commerce companies are good candidates. Logistics companies, air carriers, and anything related to supply chain management come to mind as well. But that’s just a short list. As deep learning becomes more widespread, problems of all types will be cost-effective uses of deep learning.
Large amounts of data that is descriptive of, or hypothesized to be descriptive of, the problem being solved is the first requirement to get started. People who are knowledgeable about the sourcing and lineage of the data and its meaning are also needed. Skilled data scientists with a significant mastery of neural networks are another. Large computing resources and access to neural network code and/or programming languages are other requirements. Finally, someone who has domain knowledge of the subject matter can help the development of the model and evaluate its outcomes.
Next comes selling deep learning to management. This depends on the industry and management’s technical sophistication. Many senior managers get overly excited about how emerging technologies like deep learning will solve all of their problems. Like any other artificial intelligence method, there’s nothing “push-button” about deep learning; it’s not a magic wand either.
My recommendation is to identify a few business problems where the investment in deep learning will likely produce a better result than other approaches and conduct some experiments that can produce results relatively quickly. There is a lot of hard work involved in any successful AI project. It’s best to set appropriate goals and expectations about resource needs and timelines up front.
Like all AI technologies, deep learning can be a very valuable tool for helping solve business problems. Its usefulness will grow as 1. Data becomes even more abundant than it is today. 2. Massive computing capacity continues to decline in cost, and 3. More data scientists become well-versed in its intricacies. It’s important for analytics and technology leaders to know when it’s the right tool for the job — and when it’s not.