Inequality in health outcomes in the US: Can we measure this?
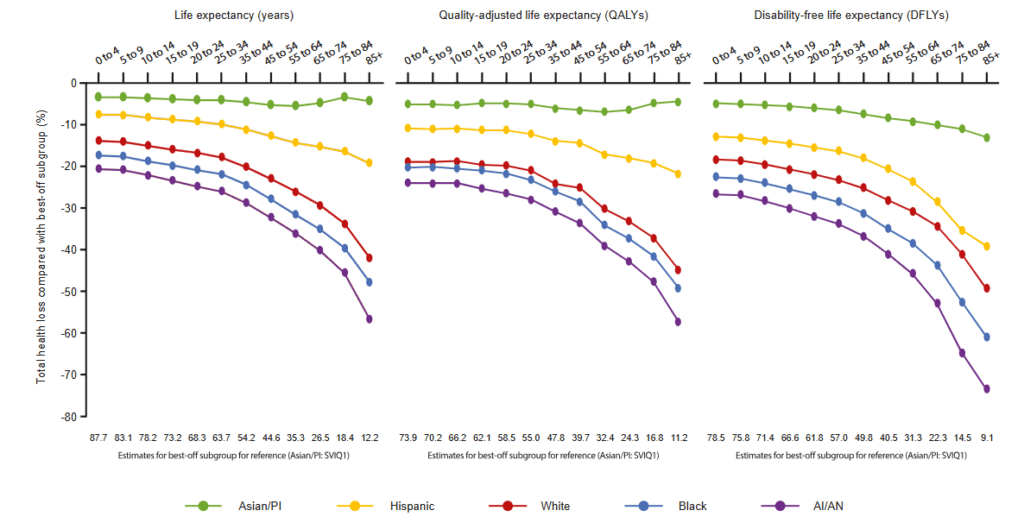
A paper by Kowal et al. (2023) does in fact measure the disparities in health outcomes. To do this, they use data from the American Community Survey (ACS), the National Vital Statistics System (NVSS) and the CDC’s Social Vulnerability Index (SVI) to estimate differences in life expectancy (LY), quality-adjusted life expectancy (QALE), and disability-free life expectancy (DFLY). The authors used a Bayesian approach to address missing (or suppressed) mortality data across race/ethnic groups (i.e., non-Hispanic American Indian or Alaska Native [AI/AN], non-Hispanic Asian/Pacific Islander, non-Hispanic black, non-Hispanic white, and Hispanic) at the county level. Mortality data came from the NVSS (via the CDC WONDER tool). Specifically, the Bayesian approach relied on a spatiotemporal models using a binomial distribution for the number of deaths by county-age group and a conditional autoregressive structure to account for county-specific effects.
Using this approach, they found that:
Life expectancy, disability-free life expectancy, and quality-adjusted life expectancy at birth declined from 79.5, 69.4, and 64.3 years, respectively, among the 20% least socially vulnerable (best-off) counties to 76.8, 63.6, and 61.1 years, respectively, among the 20% most socially vulnerable (worst-off) counties. Considering differences across racial and ethnic subgroups, as well as geography, gaps between the best-off (Asian and Pacific Islander; 20% least socially vulnerable counties) and worst-off (American Indian/Alaska Native; 20% most socially vulnerable counties) subgroups were large (17.6 life-years, 20.9 disability-free life-years, and 18.0 quality-adjusted life-years) and increased with age.
Estimated relative gaps in LE, QALE, and DFLE between the least socially vulnerable and most socially vulnerable groups, by age and race/ethnicity
These results are particularly useful as they can be used to inform baseline health inequalities applied within a distributional cost effectiveness analysis (DCEA) framework in the US. You can read the full paper here. Note that a conference abstract by Slejko et al. (2024) also estimates US-based inequality aversion parameters that you could use for your DCEA as well.